Mastering Image Labeling: Enhance Your AI with KeyLabs
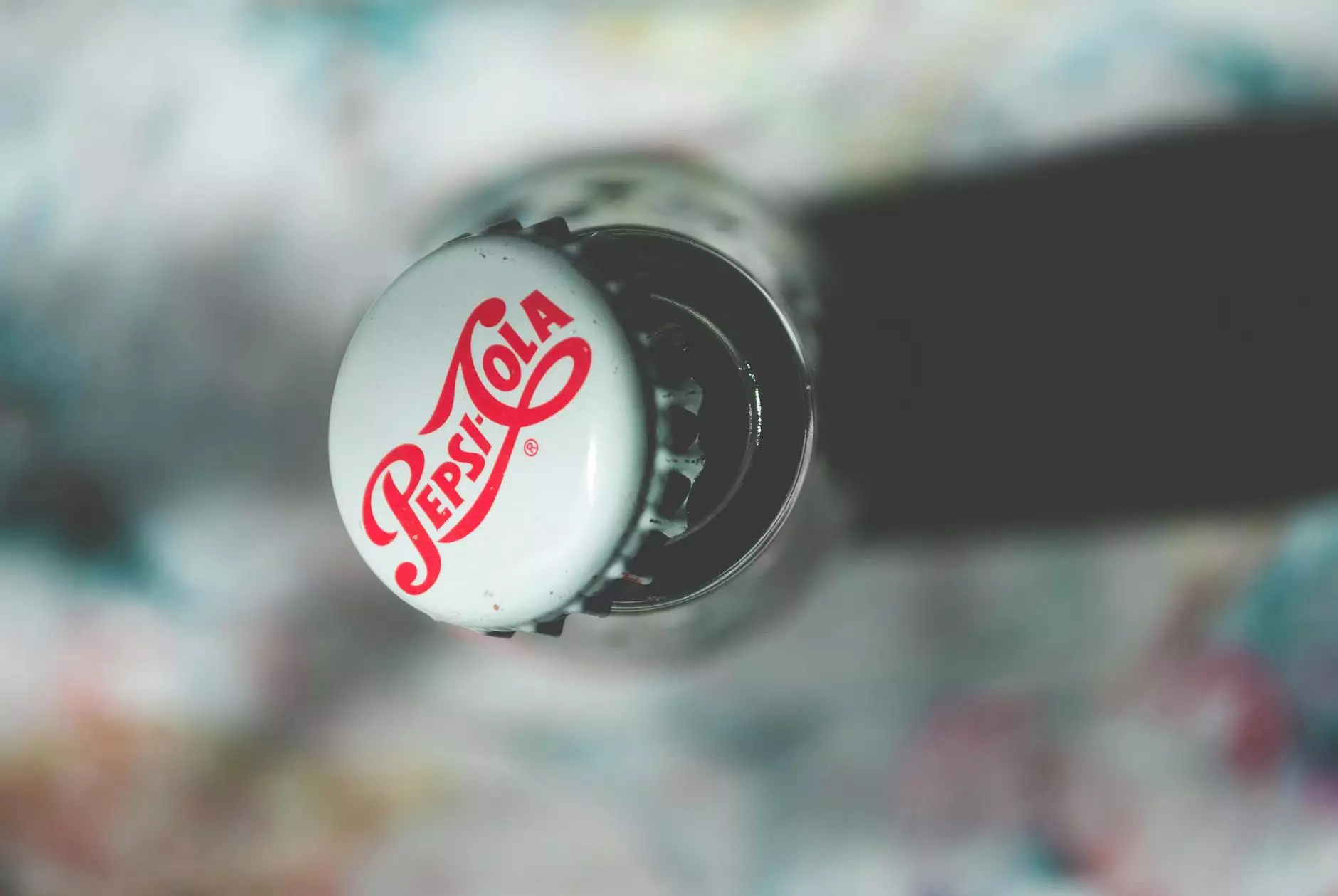
In the burgeoning realms of artificial intelligence (AI) and machine learning (ML), one fundamental practice stands out: image labeling. This process is essential for training algorithms, enabling machines to learn from visual data, and ultimately driving innovation across diverse industries. At KeyLabs, our advanced data annotation tools and platforms empower businesses to leverage the power of accurate and efficient image labeling. In this comprehensive guide, we will delve into the crucial aspects of image labeling, exploring its significance, methods, and how it revolutionizes AI applications.
The Importance of Image Labeling
Image labeling serves as a cornerstone in AI development, facilitating automatic image recognition and classification. As machine learning models become increasingly sophisticated, the need for high-quality annotated data grows exponentially. Here are key reasons why image labeling is crucial:
- Training Data Preparation: Image labeling provides the necessary training data for machine learning models. Models learn to recognize patterns and features that allow them to classify new images accurately.
- Enhancing Accuracy: Properly labeled images lead to improved model accuracy. High-quality annotations ensure that the model can differentiate between even the most subtle variations in image content.
- Application Diversification: From autonomous vehicles to healthcare imaging, effective image labeling opens the door to various applications that can be developed and refined.
- Reducing Bias: Comprehensive labeling ensures that models are trained on diverse datasets, reducing potential biases that could impact decision-making processes.
Types of Image Labeling
The process of image labeling can take various forms, depending on the specific requirements of the AI application. Here are some common types:
1. Bounding Box Annotation
This technique involves drawing boxes around objects within an image. It is widely used in object detection tasks where the goal is to identify the presence and location of one or more objects.
2. Semantic Segmentation
Semantic segmentation assigns a label to every pixel in an image, providing a more granular understanding of the scene. This method is particularly useful in applications like autonomous driving and medical image analysis.
3. Instance Segmentation
Instance segmentation combines aspects of both object detection and semantic segmentation. It not only identifies the objects but also distinguishes between different instances of the same object class.
4. Keypoint Annotation
This type of labeling focuses on specific points of interest within an image, making it crucial for applications in facial recognition, pose estimation, and other forms of detailed analysis.
The Role of Data Annotation Tools
Data annotation tools play a pivotal role in the image labeling process, streamlining workflows and enhancing productivity. At KeyLabs, we offer state-of-the-art tools designed to maximize efficiency. Here’s how:
- User-Friendly Interface: Our tools are designed to be intuitive, allowing users to quickly adapt and start labeling images without extensive training.
- Collaboration Features: With built-in collaboration tools, teams can work together seamlessly, ensuring consistency and accuracy across projects.
- Automation Capabilities: Leveraging AI technologies, some aspects of labeling can be automated. This not only speeds up the process but also reduces human error.
- Quality Control Mechanisms: Our platforms include tools for quality checking to ensure that the labeled data meets the required standards for training models.
Challenges in Image Labeling
Despite its importance, image labeling is not without its challenges. Understanding these obstacles can help businesses strategize effectively:
1. Time-Consuming Processes
Image labeling can be labor-intensive, often requiring significant hours of manual work, particularly with large datasets. Automated tools can mitigate this issue but may not eliminate the need for human input entirely.
2. Subjectivity in Labeling
Labeling can be subjective; different annotators may have different interpretations of how to label certain images, leading to inconsistencies. Establishing clear guidelines and conducting training for annotators is essential.
3. Data Privacy Concerns
With increasing awareness around data privacy, businesses must ensure that the data used for image labeling complies with relevant regulations. Implementing stringent security measures is crucial to protect sensitive information.
Keylabs: Your Partner in Image Labeling
At KeyLabs, our mission is clear: to provide businesses with the tools they need to excel in the world of AI through superior image labeling capabilities. Here’s what sets us apart:
1. Comprehensive Solutions
Our data annotation platform covers a wide array of annotation types, ensuring that we can meet the specific requirements of your project, whatever the scale or complexity.
2. Customized Workflows
Understanding that each business has unique needs, we offer tailored solutions. Whether you require fully managed services or tools to assist your team, we can adapt to your requirements.
3. Commitment to Quality
We prioritize quality above all. Our quality control processes ensure that every piece of labeled data is accurate, consistent, and ready for model training.
Future Trends in Image Labeling
As technology evolves, so too does the landscape of image labeling. Here are some trends shaping the future:
- Increased Use of AI: AI-driven tools will continue to rise, automating routine tasks and allowing human annotators to focus on more complex labeling challenges.
- Real-Time Annotation: With advancements in processing capabilities, real-time image labeling is becoming more feasible, especially in dynamic environments like autonomous vehicles.
- Integration with Other Technologies: The convergence of image labeling with augmented reality (AR), virtual reality (VR), and other technologies will create new opportunities for training models in immersive environments.
- Community Sourced Data: Platforms enabling crowdsourced annotations will gain traction, allowing businesses to tap into external resources while still retaining control over data quality.
Conclusion: The Path Ahead with Image Labeling
Image labeling will remain a fundamental aspect of AI development. As organizations strive to enhance their machine learning models, the demand for accurate and efficient data annotation will continue to grow. Embracing advanced tools and techniques transforms not only the labeling process but also the potential applications of AI.
By partnering with KeyLabs, your business can harness the true potential of image labeling and drive innovation forward. Let us empower you with the tools and expertise to succeed in the competitive landscape of AI and machine learning.
For more information about our data annotation tool and to explore our data annotation platform, visit keylabs.ai today!